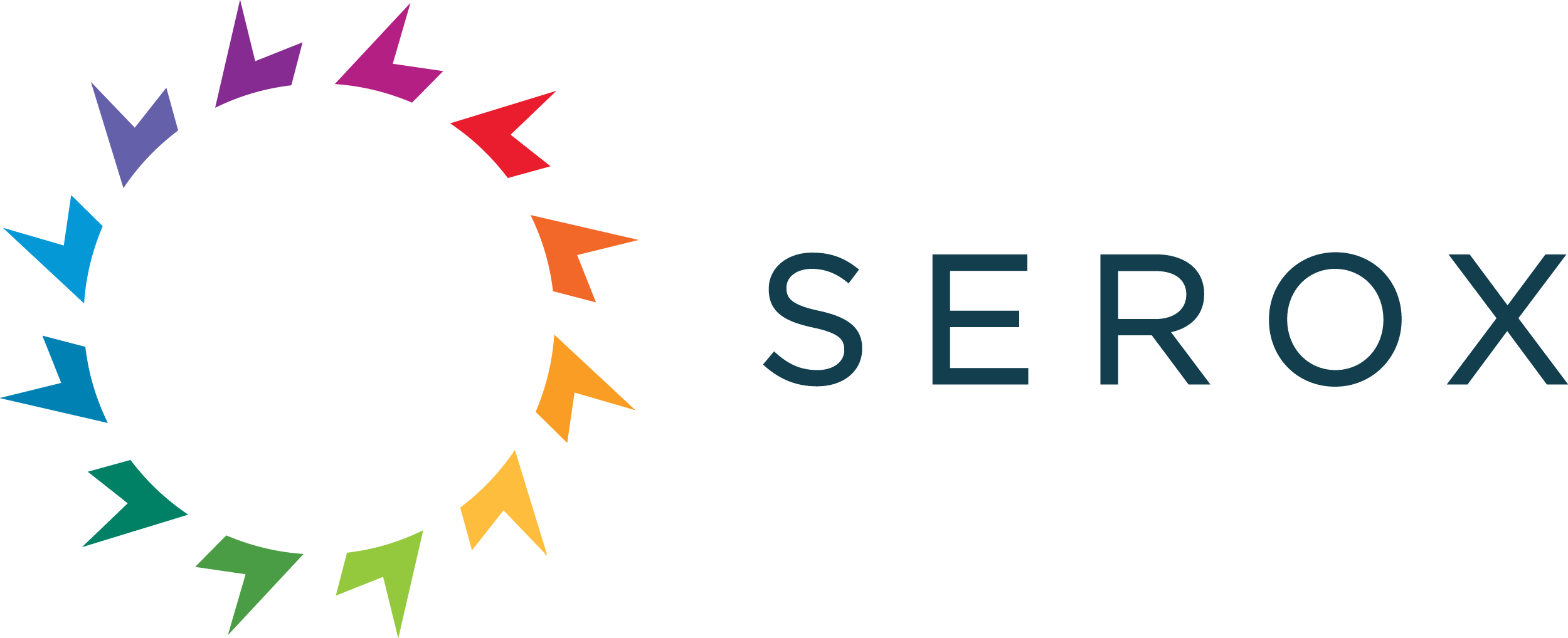
Serox are pioneering the future of diagnostics with cutting-edge technology designed to transform healthcare. This video series takes you behind the scenes of their mission, technology, and vision - showcasing how they are tackling some of the most urgent challenges in diagnostics today.
Revolutionising Diagnostics: How Our Technology Works
How can cutting-edge technology revolutionise healthcare accessibility? In this second episode, Susannah de Jager and Cici Muldoon continue their deep dive into the intersection of innovation and business strategy. This time, the focus shifts from the underlying technology to how it is applied in real-world diagnostics.
Cici explains how Raman spectroscopy, a technique that uses light to analyse molecules, is being harnessed to create a triage test for early disease detection. The goal? To make diagnosis more accessible, faster, and more accurate, ensuring that more people can be diagnosed at earlier stages. She highlights the life-saving potential of early detection—where catching cancer at stage one can increase survival rates to over 90%.
The conversation also explores the scalability of this technology, discussing how it can be integrated into existing healthcare systems and the broader vision for its impact on global diagnostics.
[00:00:00] Susannah de Jager: We've heard a bit about what technology Cici's amazing company is using, but now we're gonna hear a little bit more about how that's gonna be applied to the problem statement. Cici, thank you. How are you looking to apply Raman spectroscopy to the world of mass diagnostics?
[00:00:20] Cici Muldoon: The idea is for us to be a triage test that allows diagnostics to be more accessible for more people and allows more people to be diagnosed earlier. The importance of that is massive. If you catch cancer at stage one, oftentimes the chance of survival is 90%. If you catch it at stage four, when it's, you know, presentation at emergency department, oftentimes the chances of survival dip to 10%. So the idea is that if we could have a triage tool at point of care, it would drive the right referrals into the right further diagnostic tests.
[00:00:57] Giles Bond-Smith: My name's Giles Bond-Smith. I'm a Consultant Surgeon here in Oxford. I'm the current Clinical Director for surgery in Oxford, and my specialist interest outside of liver and pancreatic disease and emergency surgery is implementing point of care testing within the surgical field.
Early diagnosis of pancreatic cancer is key. It's an insidious, nasty cancer that creeps up unannounced and is, in the vast majority, unresectable, untreatable at the time of presentation. What Serox will hopefully allow us to do is really easily, you'll be able to give a small urine sample, pick up an early diagnostic marker that can stratify you into high risk, low risk patients, and then further investigations can be taken on. If we can crack that, you will transform one of the biggest killers in cancer today.
[00:01:53] Cici Muldoon: Imagine you wake up one day and you have blood in your urine. So you go to your GP, your General Practitioner, and you say, what's wrong with me? And they'll say, well, there's a chance this could be bladder cancer, prostate cancer, kidney cancer, or it could be UTI, it could be an STI or simply trauma.
Currently, the next step is for them to refer you for a cystoscopy. Now a cystoscopy is a very uncomfortable procedure, which causes anxiety in patients and carries a risk in which a camera is put into your urethra. Now you can imagine that nobody wants to have this.
[00:02:32] Susannah de Jager: No.
[00:02:33] Cici Muldoon: It also caused the NHS a thousand pounds per procedure and in the states a cost between, $1,600 and up to $15,000 for each procedure.
Now, the crucial thing about these cystoscopies, is that 80% of them are negative. So 80% of those tests didn't need to happen, and you can see why, as a doctor, you would want to refer the person away just in case. You want to rule out the possibility that they might have bladder cancer, so you refer them away.
But nevertheless, it's a cost to the system. It's a source of anxiety for the patient. So if you could have a test that happens at point of care before that referral happens, if you could pee on a stick, put it in a spectrometer and in sub-three minutes have a red, amber, green answer that says, you definitely need to be referred away for cystoscopy, or you do not. That would be a fantastic thing.
[00:03:25] Scott Sherwood: I'm Scott Sherwood and I'm a board member at Serox. Serox's technology, you can do a number of different diagnostics, and get results on the spot in three, four, maybe five minutes tops. So you're improving workflow, you're becoming much more efficient and you're not, you know, you're making the appropriate referrals and you're hopefully eliminating a lot of unnecessary procedures like the cystoscopy, example.
[00:03:52] Ben Turney: Hello, my name is Ben Turney I'm an Associate Professor in Oxford University and also a Consultant Urological Surgeon in Oxford.
So one thing that I think we're all familiar with is that people want immediacy of testing, and what we really need is patient testing that can happen at the point of care that patients then are reassured that, either everything's okay or actually that they need to proceed to further diagnostic tests.
So the key thing for something like Serox's product is that this could be in the clinic. We can take a sample immediately from a patient and hopefully within a few minutes give the patient either some reassurance, everything's all right, or a diagnosis, which may lead to other further investigations or institute treatment rapidly.
[00:04:30] Susannah de Jager: You spoke already about how Raman spectroscopy has been used in urine analysis before, but why hasn't it been applied to this solution? Why hasn't it been taken out of the lab?
[00:04:43] Cici Muldoon: The question you're asking is why now? The why now is because the cost of the components is coming down. So the cost of lasers and detectors has dramatically dropped in the last 10, 15 years, and the advent of machine learning is really what unlocks the problem.
So AI, as most people refer to it, allows us to see patterns in the data that we would not be able to have seen previously, and we can extract a huge amount more information from the data that you see. So essentially, a drop in component cost plus AI is turbocharging the field of spectroscopy and allowing it to be applied in a healthcare setting.
[00:05:21] Peter Dobson: Interestingly, the Raman spectroscopy process has been looked at a little bit in the past, but I don't think it has been taken so seriously because of sensitivity issues, but now with the Surface-enhanced Raman, and especially if we use the right way of enhancing selectivity, I think there are some unique opportunities and we are exactly in the right timeframe to do that.
And of all the ideas I've heard for AI and machine learning. This is one which really looks as though it's viable. It's often used as a way of winning extra money, but in this case it is obvious. This is where you can make use of a library of information, which is unique in this case, compounds in urine, and you can detect them in a new patient and compare them with this large library. This is what AI machine learning's all about, and I think this is the thing that really pushed me into saying, yes, I wanna work with this team.
[00:06:28] Susannah de Jager: Cici, the argument you make is so compelling. Can you tell me a bit more about how you're using an existing body of evidence around Raman spectroscopy, photonics and machine learning, and what's innovative about how you're applying it?
[00:06:44] Cici Muldoon: So a huge part of the innovation here comes in taking this technology out of the lab and into a commercial setting. Normally, these experiments happen in a controlled environment with a big spectrometer in the corner of a temperature controlled lab used by an expert operator. To be able to take this type of hardware out into the field and have different machines that can compare results is completely non-trivial.
[00:07:15] Susannah de Jager: So it's exactly like computers. They used to take up a whole room, they're now smaller. They've gone into, in our cases, our homes. But you are talking about into the doctor's office.
[00:07:24] Cici Muldoon: Yes, and the question here is not just one of usability. So this has got to be a test that is very simple to use by a non-expert operator, but it's also about calibration.
You have to be able to make sure, particularly when you have these machine learning based approaches, that the data that you're getting from spectrometer one is comparable with the data that you're getting from spectrometer 2, 3, 4, 5, 6, 7, 8, 9, 10. So we're very proud that we're working with the NPL, the National Physics Laboratory on this problem of calibration.
We have completed one project six months, and onto the next one. Surrounding the calibration of these spectrometers and how you can incorporate all of that calibration into our cartridges with them.
[00:08:05] Susannah de Jager: I'd love to get a bit more detail of the cartridge itself.
[00:08:08] Cici Muldoon: The cartridge is there principally as a carrier for our nanoparticle substrates, which are what we use to tailor the test to one disease over another and to amplify our signal.
However, we've also got patents around calibration being worked into the cartridge and around sample preparation being worked into it. So there's quite a lot that goes into the cartridge.
[00:08:31] Susannah de Jager: And there's a lot of defensible IP there. You know, that's part of the moat that you're building. That means not everyone can just jump on this.
[00:08:37] Cici Muldoon: Without wanting to give away too much. Yes, the cartridge is where most of our IP is. The machine learning is, sort of secret sauce, and the hardware is really just, it's off the shelf components and it's about the sample presentation and that's, you know, a sort of engineering problem, if you will.
[00:08:55] Susannah de Jager: Amazing. So this is an established technology, but what your company has done hugely successfully and in collaboration with NPL is bring it out of the lab. Put it into machines that are calibrated and you've created unique IP within your cartridges.
[00:09:09] Cici Muldoon: And also the important thing here as well is that we are thinking commercially. So it's not just about the technology and how great it is and whether it can be applied in this field. It's about how it can be brought to market. I think this is where a lot of great technologies fail because you don't think about the pathway to market and the costs and whether it's feasible.
So a lot of our thinking has been around how do we leverage existing, for example, lateral flow test, manufacturing capabilities in order to use our surges substrates on an LFT base essentially. We're thinking about how we can outsource, manufacture and distribution of spectrometers, how we can use off the shelf parts for the spectrometers so that we don't reinvent the wheel, and so that we have a quicker pathway to market, because that's really important. So commercialising the technology is not just about finding clever ways to make sure that all the spectrometers are calibrated, but finding ways in which this can be done in a commercially feasible way.
[00:10:14] Susannah de Jager: So historically this area hasn't been successfully pioneered into with machine learning. Can you talk about some of the ways in which you are overcoming those historic challenges?
[00:10:25] Cici Muldoon: To date, most diagnostic tools are expected to have a quantitative outcome. Now, there's a bit of a fallacy here, because if you get an x-ray in Mexico City on a Phillips machine and you get an x-ray in London on a Siemens machine, you're gonna be comparing results from two different machines. The scales might be slightly different. The interpretation of the doctor might be slightly different. So this idea of ultimate quantitative answers and existing diagnostic modalities, you know, is not exactly true.
However, there is an understandable hesitation to accept a black box machine learning outcome where you come in one side and you say, I'm gonna feed you the signal from all these biomarkers and I'm gonna come out the other and get a classification. So what we are trying to do is say, okay, we can zoom in on certain parts of the spectral response and look at the ratio of two things. So we, it's essentially using machine learning, and more quantitative approaches at the same time, and finding ways to innovate that would allow machine learning to be more acceptable to a regulator.
[00:11:30] Susannah de Jager: Cici, thank you so much. That's a really helpful summary for me as a lay person on how you're going to be applying these amazing technologies to the world of mass diagnostics, and it sounds just so compelling and important.


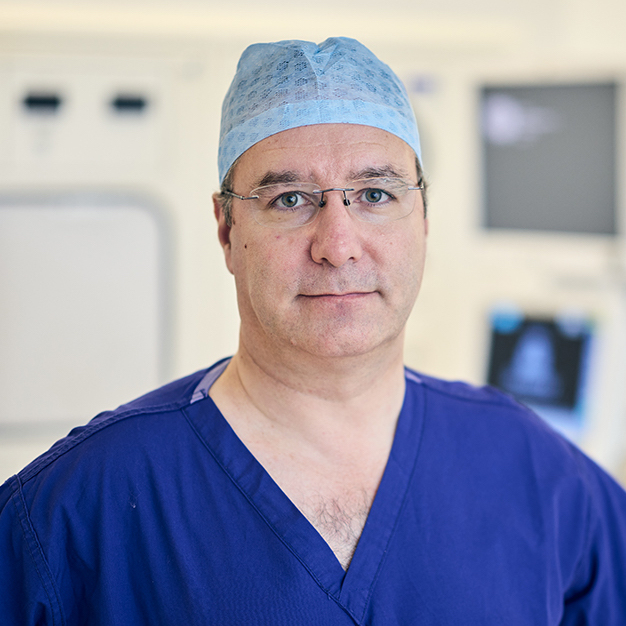
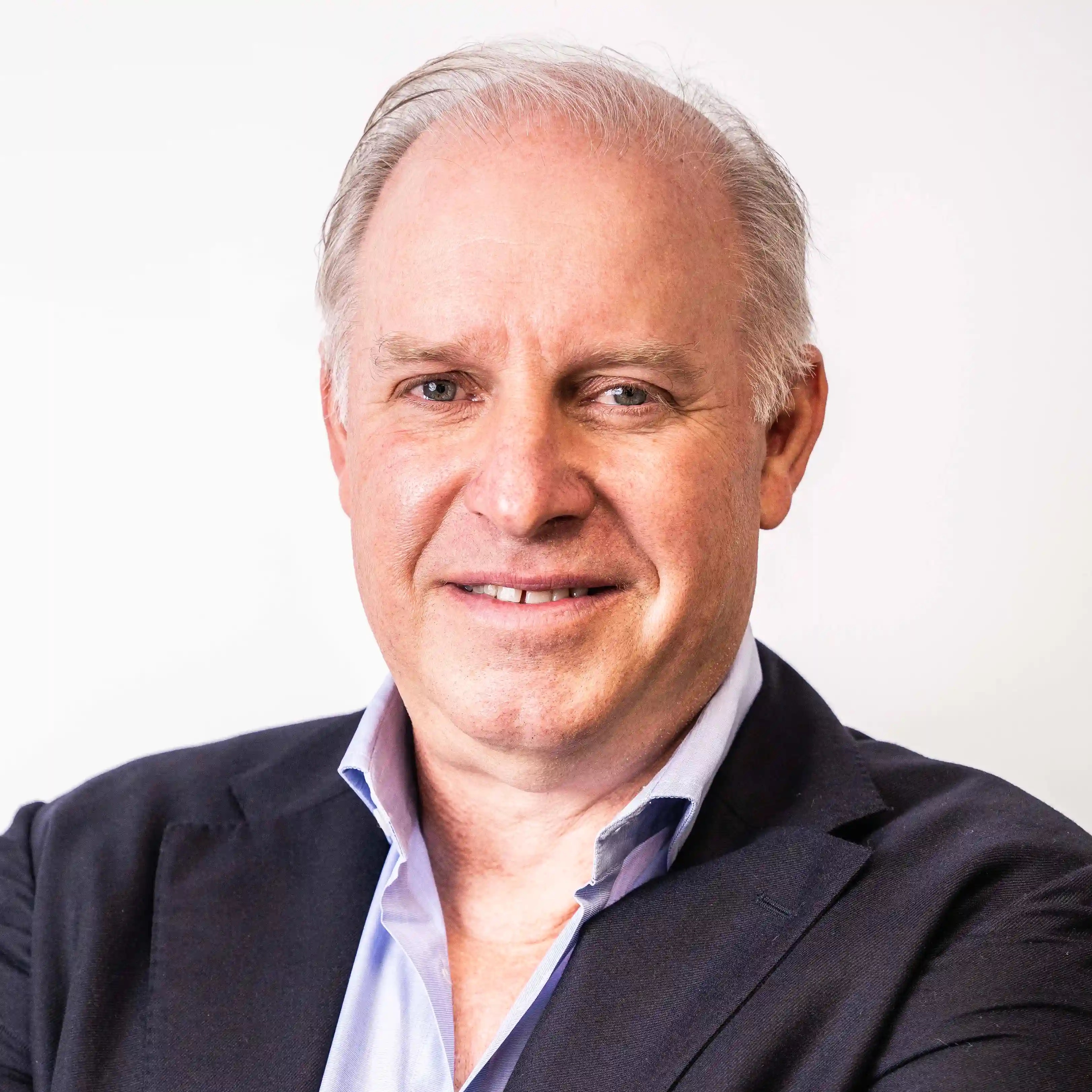

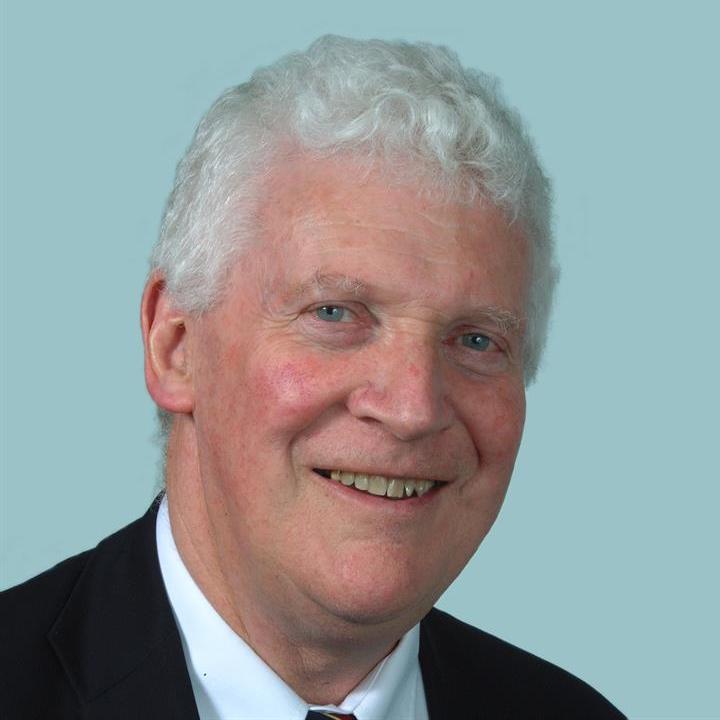